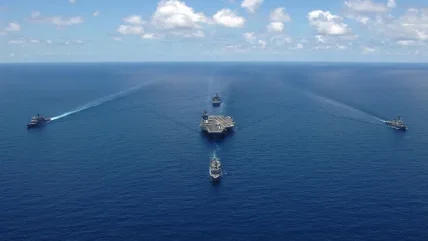
Given the deteriorating geopolitical climate, defence budgets coming under strain due to inflation and the ongoing supply of military aid to war-torn regions around the world, the $64,000 question is how Western navies measure up when it comes to combat readiness. The short answer – they could be readier.
As the US Government Accountability Office (GAO) laid out in its December 2022 report, ‘Military Readiness: Actions Needed to Further Implement Predictive Maintenance on Weapon Systems’, the US Department of Defense (DoD) “is continually challenged to provide battle-ready ground combat systems, ships and submarines, and aircraft to its war fighters, spending nearly $90bn each year on weapon systems maintenance”.
The US Navy serves as a case in point, with a 2018 report by the GAO – ‘Navy Readiness: Actions Needed to Address Costly Maintenance Delays Facing the Attack Submarine Fleet’ – demonstrating that between fiscal years 2008–2018, its fleet of attack submarines alone incurred 10,363 days of idle time and maintenance delays as a result of slowness getting into and out of shipyards. An extreme example was the USS Boise, a Los Angeles-class submarine, which was due to enter a shipyard for an extended maintenance period in 2013. Due to heavy shipyard workload, the sub saw its maintenance period considerably delayed. By June 2016, it could no longer conduct normal operations and subsequently remained idle pier-side for over two years, waiting to enter a shipyard.
Embrace the algorithm
Much of the US Navy’s shortcomings in this area can historically be put down to bad management and bad planning. However, the DoD has recently grasped the nettle – given the need to improve maintenance turnarounds – by employing condition-monitoring technology such as sensors, data analytics, algorithms and AI to schedule maintenance based on evidence of need. In short, it has finally chosen to embrace predictive maintenance. The argument is that, if implemented correctly, predictive maintenance can reform a military’s approach to weapon systems readiness by reducing unplanned and unneeded maintenance, cutting maintenance delays and shaving sustainment costs, according to officials.
Already, the US Navy has predictive maintenance pilot projects in place for surface ships – including USS Mason, which began in 2020, and USS McCampbell and USS Bulkeley, which both started in 2022. These pilot projects aim to test proof of concept and further develop analysis techniques, as well as determining how best to provide predictive maintenance prompts to uniformed maintenance personnel aboard US ships.
In 2022, in the same vein, the US Navy issued guidance requiring the use of predictive maintenance on all new and existing surface ships, submarines and aircraft carriers, where technically feasible, costeffective and beneficial. For Tiedo Tinga, professor of life-cycle management at the Netherlands Defence Academy and professor of dynamics-based maintenance at the University of Twente, defining ‘predictive maintenance’ is a relatively simple affair. “My definition of predictive maintenance is [that it’s] a form of preventive maintenance, for which the future optimal moment of repair or replacement of the system or component is based on a combination of the present condition and a prediction or calculation of the remaining time to failure.”
Predictive maintenance can be considered an extension of condition-based maintenance, which bases decisions on the present condition of the system under review. Condition-based maintenance disregards any future degradation, and as a result frequently requires immediate action when a condition threshold is exceeded. Predictive maintenance provides additional benefits in adding response time, allowing for proper organisation of spares, facilities and workforce for upcoming maintenance tasks.
Within predictive maintenance solutions, AI and machine learning (ML) constitute one approach to obtaining the remaining useful life (RUL) out of a system. Alternatively, applying physics-based degradation models – covering, for example, wear, fatigue or corrosion – is another approach that can be followed. The advantage of using AI and ML is that only limited system or domain knowledge is required, as the algorithms simply analyse the data to obtain the required relations and predictions.
The limited data sets challenge
That’s not to say that predictive maintenance doesn’t face obstacles. “The disadvantage is the large amount of high-quality data that is required to train these algorithms,” says Tinga. “Specifically, [data related to] a considerable number of actual failures is required. That is a big problem in maintenance in general, and in military sustainment in particular, as maintenance of critical systems aims to prevent failures.”
Typically, the number of examples for training AI is limited, or the examples are associated with a completely different operating profile of a ship or system, which makes it less useful, according to Tinga. Yet, there are successful examples of AI in maintenance – today, these are mainly related to fault or anomaly detection or diagnostics. However, AI shouldn’t necessarily be viewed as a magic bullet. Indeed, the largely variable and unpredictable operation of military systems makes it more difficult to effectively make use of. AI is far easier to train with known, repeatable processes, but it works less well in uncertain settings where situations appear that haven’t previously been seen before, according to Tinga.
As Tinga explains, the Royal Netherlands Navy is now focusing on ensuring that relevant and highquality data is collected and properly stored for a wide range of systems and installations on board, in order to create a long-time historic data set. At the same time, it is also exploring methods and algorithms that could be used for practical applications, such as fault detection and monitoring of usage profiles. It is heavily involved in two projects in the European Defence Fund – EDINAF, which concerns digital ship architecture, and dTHOR, which focuses on ship structural health monitoring. Looking ahead, Tinga expects to see navies increasingly invest in the ongoing development of digital models in the design and build process of new ships, which in the future may allow for the greater use of configuration management and predictive maintenance. For existing ships, however, this is still far away, so efforts need to focus on collecting pieces of data that can be directly beneficial for specific maintenance issues. “Rather than collecting everything, the focus should be on identifying specific issues and finding dedicated ways to add sensors and collect relevant data,” Tinga notes.
$90bn
The amount spent by the US DoD each year on weapon systems maintenance.
“In our research, we strongly focus on analysing data collected by dedicated condition monitoring techniques and sensors – vibration monitoring, motor current signature analysis – rather than data from more general process or control-related sensors – temperature, pressure – as the latter are much more indirectly related to degradation and failure,” he adds.
13,363
The number of days between 2008–2018 that the US Navy’s fleet of attack submarines spent either in idle time or maintenance delays.
GAO
Increasing adoption
While the jury is still out regarding AI – at least in the short-term – practical AI solutions are gaining traction nonetheless. Case in point is a March 2023 agreement between Gecko Robotics and the US Navy. Under this deal, Gecko will use its wallclimbing robots and AI-powered software platform to build digital models of the vessels in order to increase the speed of maintenance cycles and reduce the time navy vessels spend in dry-dock. The expansion of this work follows the approval of Gecko’s Rapid Ultrasonic Gridding (RUG) process by the US Navy.
RUG creates data-rich visual grid maps identifying areas where corrosion and other damage mechanisms have caused wall-thinning. When incorporated into a risk-based inspection (RBI) programme, it improves the ability to visualise the state of mechanical assets.
In the case of Gecko Robotics, its robots rapidly capture up to 176 readings per foot at maximum speeds of 60ft per minute, thereby enabling it to quickly inspect large sections of an asset and provide data density regularly exceeding a million A-scan data points. As a result, inspections are completed in a few days, rather than over many weeks.
The French Navy has similarly been making moves in this area by testing numerous measurement drones underwater for hull inspection. However, for the time being, these are seen as being a complementary means of assisting decision-making and gaining access to hard-to-reach places. A spokesperson for Marine Nationale, Service de Soutien de la Flotte – otherwise known as the French Fleet Support Service (SSF) – highlights that predictive maintenance isn’t an inherently new concept, arguing it has been decades since maintenance on electric engines, based on vibration measurements, or oil change with oil analysis, has been in operation.
“It was a predictive maintenance based on a single piece of data. What is new today is data quantity and connectivity,” the spokesperson explains. “Any new equipment comes with dozens of real time instrumented parameters. [As a result,] computers and software have the ability today to do whatever we can imagine with them.
“We have acquired two remotely operated vehicles (ROVs) for walls of wharf and caisson gate inspections; either for ships’ operational needs or for inspection; [but] we are at the very first step in terms of getting used to those tools and including them in the normal procedure of monitoring.”
And therein lies the point; the technology of ship design needs to catch-up with AI – and for major datasets to be established for the latter to be able to fulfil its potential.
“For now, we have numerous embedded monitoring solutions, the issues being to synchronise data and have a shared and easy access,” the spokesman concludes.
It’s clear that, regarding predictive maintenance, the onus is now on modern navies to look forwards, not backwards. AI and ML are rapidly developing and look set to assume greater importance longer-term, as long as ship design keeps pace accordingly – otherwise historical problems will be more difficult to overcome. AI and ML need large amounts of data. For navies, the challenge is on.